I just finished my Australian Road Show with the Web Directions folks. It was really illuminating doing the workshop three times in a row. I conduct five classroom exercises in each day-long workshop, and one thing really stood out for me this week. When workshop attendees tried their hand at grouping data (represented by a deck of cards with verb + noun labels on them) by affinity, things sometimes fell apart in two different ways.
The first part of the problem was because the data I gave them to group has to do with training for marathons, which, to my chagrin, is not widespread in Australia. By the last workshop, I managed to alleviate misinterpretations of the data by explaining what it all meant up front. However, the folks in the Canberra workshop really struggled with marathoning concepts. (Sorry for that!)The second, more striking, observation I made is that workshop attendees often tried to group too high by making a few labels like “prepare,” “monitor,” and “track,” then putting all the cards into these three or four big broad categories. Each category would have 20 cards or so. Then the groups would try to investigate each of those piles of cards for more detailed subsets and encounter difficulty.
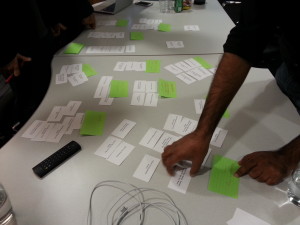
(Sorting marathon cards at the Melbourne workshop.)
That’s a top down approach. Even though I asked them to work from the bottom up, to refrain from putting their own model of the data together at the top level, to avoid making boxes and sorting stuff into them, they did this. I don’t think the groups realized they were working from the top down. They were thinking it was more of a way to break down the large amount of data into more manageable piles. I guess it is a natural tendency for many of us. In our own process, if we have a lot of data, we want to break it down into a few sets and attack each set separately. It reduces cognitive overhead, if you will, and makes us feel less overwhelmed.
Truly the best way of grouping data into subsets from the bottom up is to randomly select one piece of data to begin with and compare it to all the other data to see what is like it. Ask yourself, “What does this person intend by saying this?” What’s behind a label like “Stop to Remove Rock from My Shoe” or “Enjoy the Fall Colors?” For the first one, the intent is to make my running as comfortable as possible, since it is quintessentially an uncomfortable process. The second label represents the intention to get a spiritual boost from the run, perhaps associated with distracting myself from the pain. By looking at the intent behind a label, workshop attendees found it much easier to find similarities between cards spread out on the table before them.
I figured these observations were important enough to share with the general group. We’re all data analysts of one stripe or another. It helps to remind ourselves that the data needs to be assessed one droplet at a time, rather than as a set.